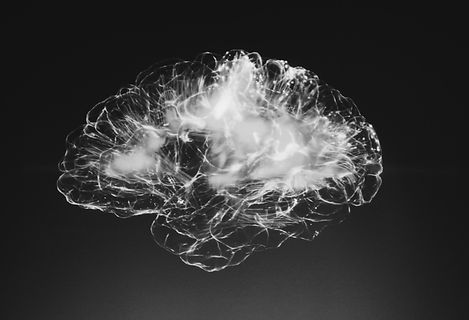
Research
Computational and neural mechanism of social learning
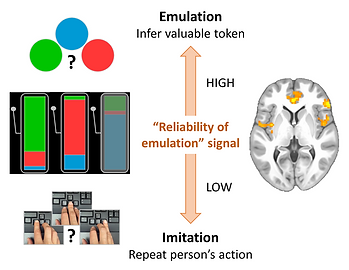
We are interested in understanding the mechanisms underlying how people learn in social contexts. This can involve observational learning (learning from observing another person), learning about another person (for example, learning their preferences and attitudes), social influence (how does other people’s preferences and attitude influence one’s own), and strategic learning (think of any two person game where one’s choices affect the other player and vice-versa). We study those mechanisms by developing carefully controlled behavioral tasks, where the specific sequence of events can induce learning through different strategies, then we apply computational models, derived from reinforcement learning and Bayesian updating, to understand exactly what computations people perform when solving these tasks. Finally, we also study participants’ brains while they are performing these tasks in an MRI scanner, so we can test whether and where those computations are represented in the brain. In the future, we plan to study how those social learning processes take place in more naturalistic contexts, for example through live social interactions between several members of a group.
Relevant references:
- Charpentier, Iigaya, O’Doherty (2020) Neuron
- Charpentier & O’Doherty (2021) Book Chapter in The Neural Basis of Mentalizing
- Charpentier et al (2014) Journal of Neuroscience
An ongoing research project, funded by a K99/R00 award from the National Institutes of Mental Health (2021-2026), aims to investigates how the behavioral and neural computations involved in social learning vary across individuals, in particular along psychiatric symptom dimensions, such as social anxiety, and along the autism spectrum and neurodiversity more broadly. In this project, we are moving beyond the traditional “one-task-one-model” approach by (1) collecting multiple tasks assessing different aspects of social learning within the same individuals and (2) considering heterogeneity in strategy use during computational modeling analyses. Our recent data suggest that these approaches may help better understand and characterize the heterogeneity in autism-like traits and/or better dissociate psychiatric symptom dimensions that tend to be strongly associated in the general population and in the clinic.
Relevant references:
- Charpentier et al (2023) preprint: https://osf.io/preprints/psyarxiv/pcjg7/
- Wu et al (2023) preprint: https://osf.io/preprints/psyarxiv/mfc7z/
Variations in social learning computations along psychiatric symptom dimensions and neurodiversity
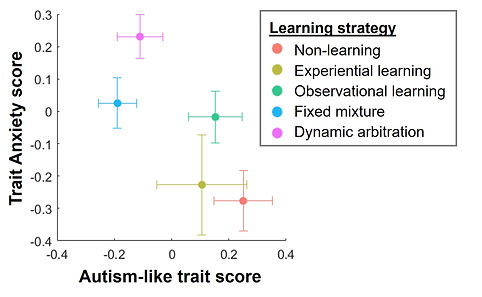
Information-seeking in social contexts

As humans living in a socially connected world, we constantly have to decide whether to seek or avoid information out of the unprecedented amount of information available to us through online and social media. We have been studying the factors that influence how people value and select or filter out information, in particular the combined effects of hedonic motivations (preference for desirable information, even when it provides no instrumental benefit) and of cognitive motivations (desire to reduce uncertainty). We are also interested in studying these processes in social contexts, for example when people seek information about other people, or information about themselves from other people. Ultimately, understanding these processes could shed light on disrupted patterns of information-related behavior and their impact on society and on mental health.
Relevant references:
- Charpentier, Vellani, Cogliati Dezza, et al (2022) Scientific Reports
- Charpentier, Bromberg-Martin, Sharot (2018) PNAS
Human cognition is biased in ways that deviate from optimal behavior. In particular we are interested in using a combination of tasks, surveys, computational models, naturalistic data, in the general populations and groups with societal or clinical relevance. We have studied economic decision-making biases, such as risk and loss aversion, in the context of emotion processing and anxiety. Currently, we are working on quantifying confirmation bias, that is the tendency to weigh social information that confirms prior beliefs, and plan to extend this approach to other biases (e.g. blindspot, false consensus, reactance bias). We hope to provide a better characterization of the individual susceptibility to biases in information processing and belief formation, and its variations across individuals and groups.
Relevant references:
- Charpentier, Zbozinek, et al (2021) Computational Psychiatry
- Charpentier et al (2017) Biological Psychiatry
- Charpentier et al (2016) Social Cognitive and Affective Neuroscience
Characterization of cognitive biases during social and affective decision-making
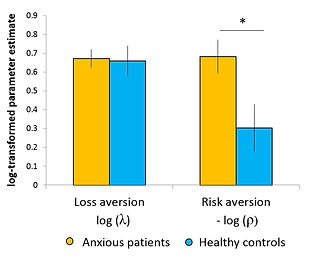